What is the Future of Generative AI in the Enterprise?
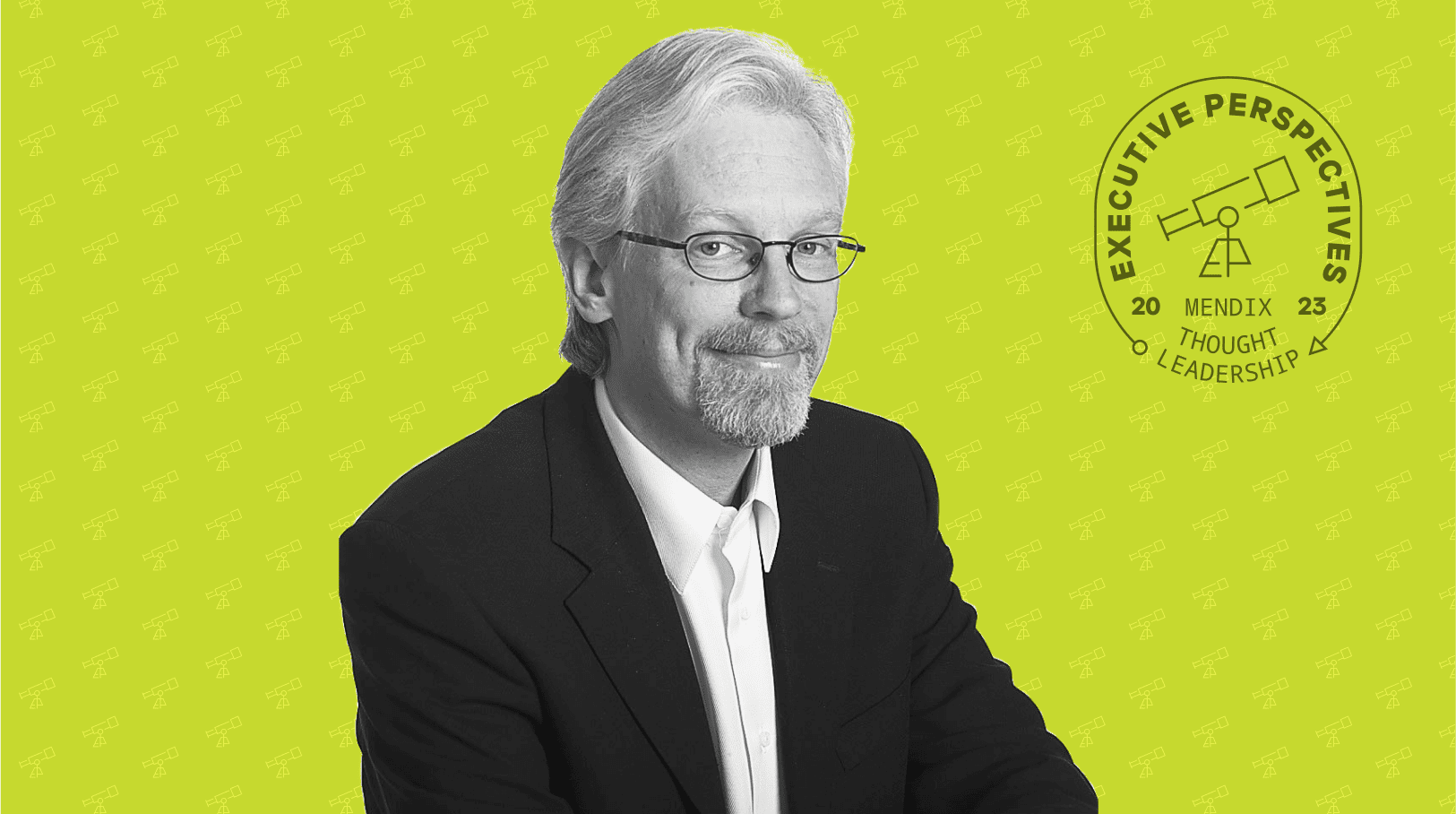
I’ve seen many hype cycles. (In fact, as a former analyst, I’ve contributed to and overseen the creation of a few).
Gartner aptly defines a Hype Cycle™ where you experience the promise of a certain technology or the application thereof. Then comes a period of disillusionment, followed by rejection or acceptance, then (quite often) productivity.
Outside of the analyst realm, however, the world is seeing a cycle of artificial intelligence (AI) hype spinning up again with the buzz around ChatGPT and generative AI more broadly. I won’t attempt to predict the future and tell you where AI will be in ten years. What I can tell you is that AI’s use in the enterprise will continue to grow and evolve—ChatGPT or no ChatGPT—and you need to harness it so you can reap its benefits for years to come.
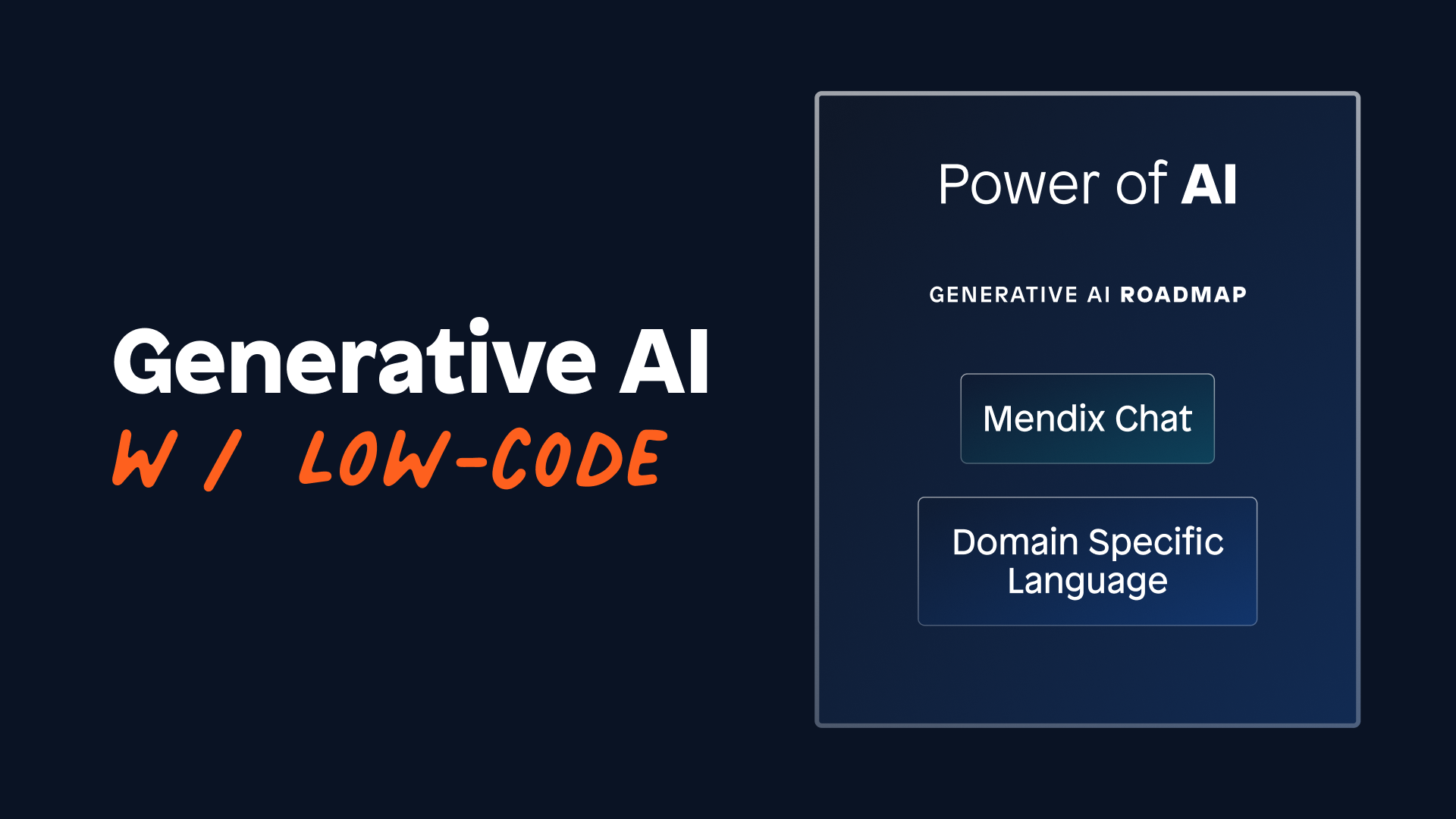
Is generative AI the one AI to rule them all?
In the past, the difficulties around designing artificial intelligence and implementing traditional machine learning prevented AI from being implemented in the enterprise. It was a lot of work to get a corpus of data, prepare it, build the model, train it, and then put it into production. Let’s say you were successful at that. That only meant you needed to expend more energy and resources maintaining the model and dealing with the various forms of “drift” that can make it less accurate and effective over time.
With computational power increasing and data sets getting larger, AI in the enterprise is growing. According to Forrester analyst, Diego lo Guidice, implementation of AI, machine learning, or deep learning has gone up from 67% in 2021 to 73% in 2022.
I’d even argue that AI adoption is closer to 100% as of this writing. However, it’s a matter of what “adoption” means here. “Traditional” artificial intelligence is already, of course, fueling things like
- recommendations across a wide variety of use cases
- automated document processing
- algorithmic trading
- supply chain planning
- cybersecurity
The primary applications of machine learning right now make routine work easier and, for the tasks that are easily automated, are faster at it than people are. Such forms of AI are rapidly becoming ubiquitous in every-day work lives. No doubt they offer value of speed, time saved, compliance met, and so on. But these are now tablestakes for enterprises.
This new wave of machine intelligence represented by generative AI – despite how truly “intelligent” it may or may not be – has clearly sparked a massive amount of exploration and investment. And for good reason. ChatGPT and other generative AI tools like Bard or Dall-E are technical marvels.
Generative AI has the potential to unlock significant value and it has huge implications for all forms of work. It’s tempting to think that because it embodies such a phenomenal amount of data and can do such astounding things, that generative AI will supplant other forms of machine intelligence. That all it takes is some clever “prompt engineering” and some deft “fine-tuning” to generate a solution to any problem. Why not, right?
ChatGPT can accelerate human tasks. I heard a story recently of a software tester who wears a number of hats managing a small New England farm’s IT operations. The farm’s management asked the tester to find a particular IoT solution for their hydration system. In what would normally be a task that could take several days, the tester used ChatGPT to create a list of nearly 60 requirements in a few hours. He was able to combine his knowledge and esoteric experience in the agricultural IT world with generative AI to hasten his work.
However, generative AI can’t do it alone.
Generative AI can augment knowledge workers. A legal firm, which produces myriad legal documents all day long, could possibly use generative AI to produce documents bespoke to the case it’s related to.
While hours saved in these examples is beneficial, you’re still only making routine work easier. There’s more value to wring out of AI.
In a recent article by Somnath Singh, “Bill Gates: People Don’t Realize What’s Coming” Singh posits that this new wave of AI, spurred by generative tools like ChatGPT, will soon change how enterprises solve business problems.
Singh puts forward to his readers the idea of “a world where the boundaries between technical and non-technical work no longer exist.” This is no doubt true. People will need less technical skill in a particular area to do their work and accomplish their goals.
However, generative AI can’t do it alone.
Composite AI: Better together
If we take a step back, it’s useful to remember that artificial intelligence is a broad term. And as with all nascent technologies, the buzz associated with any particular form of AI should be taken with a substantial grain of salt. You need to ask yourself which flavor of AI is being talked about. This helps you identify if a particular AI technology or technique can be put to use to solve a particular challenge or unlock an opportunity for your organization.
Solving a problem through machine intelligence is nearly always multifaceted in practice. And no matter how rich or inspiring a given AI technique is (and generative AI is surely rich and inspiring), it only addresses one facet of augmenting or automating work through AI. So, if you’re aiming to solve a real problem with artificial intelligence, you likely need an amalgamation of AI techniques, not just one. Or, as Gartner puts it: Composite AI.
Composite AI brings multiple AI and advanced analytics capabilities together, with the goal of producing a better — and more trusted — result. For example, a knowledge graph is often used as a complement to generative AI when augmenting or automating human decision-making, or when interacting with virtual agents. As the name suggests, it’s a great way to capture human experience and judgment. Infusing the human factor, in digital form, drives better outcomes. And it increases user confidence in system-generated responses to their questions.
Here’s an example of composite AI: There’s an application that allows an insurance company’s customers to snap a photo of their car after an accident. From that, an assessment of the damage is automatically created. Based on that assessment, a report is produced. And if the car is deemed totaled, a call to the nearest towing company is initiated for the car owner.
The insurance organization in this example is using a number of AI frameworks to automate manual tasks as well as human decision-making. Image recognition captures and assesses the damage. Machine learning compares the damaged vehicle to what a vehicle of that make and model should look like and determines the damage. Generative AI produces a report. This composited AI can then deem the car totaled and determine the course of action of calling a towing company to pick up the car.
Causal and effective
This leads me to one of the more interesting forms of composite AI: Causal AI.
Causal AI is more than generative AI or machine learning predictions and automation. Those technologies don’t have an understanding of concepts; there’s no discernment. Causal AI can analyze input and—provided the model is trained properly—make human-like decisions. With causal AI, you can capture your employees’ judgment within certain systems or workflows. With this, you’re not just automating a task. You’re improving decision-making.
With causal AI you can level up professionals’ abilities and arm them with the ability to make better decisions faster. With time, you could even trust that model with a class of decisions. With the right data set—and trusting it with a certain class of decisions—organizations can now augment their whole workforce with better and faster decision-making abilities.
It’s still a few years away, but it’s the next step in AI. Imagine the value you can realize with technology like that. Causal AI is more than hours saved and dollars earned. It’s more than forecast prediction. It’s forecast prediction along with recommendations for suggested sales tactics for certain customer segments. For instance, causal AI can analyze stock market data and help financial institutions make informed investment decisions based on the relationships between stock prices, economic indicators, federal policies, and news headlines.
Whereas an organization can use machine learning to automate a task along a supply chain process, causal AI doesn’t just replicate a human task, it can replicate human decision-making and identify bottlenecks and inefficiencies in the process. Not only can it identify, it can also improve performance.
Causal AI is another way to create better outcomes.
Causal AI, and other implementations of composite AI, are going to help you productively blur the boundaries of technical and non-technical work, so you can start to see the return on investment you put into the development of AI-enabled applications and smart apps start to grow exponentially.
Get hyped
“Technology in all its consequences means a persistent change.”
The promise of technology, F.F.P Kollman
AI will always be pushing itself forward, changing the game it just changed. Understanding its consequences and the value you can gain from it is how you always push your organization forward. To me, the excitement of AI is not what it can replicate but what we can do with it, and what sort of complex problems we can solve.
When hype around AI starts to reach a fever pitch again, it may very well be about AI that can apply human judgment while lowering the technical expertise required to perform work. Thusly, careers will change, job roles will be altered, and industries will be disrupted.
But that’s the nature of technology, isn’t it?
It’s up to you to determine which AI technologies, not just a singular heavily hyped one, will help you maintain your organization’s viability.